AI: Bridging the Gap in Value-Based Mental Health Care
As healthcare continues to shift towards value-based care (VBC), organizations and health systems are prioritizing patient outcomes and cost efficiency over traditional volume-based models. However, the adoption of VBC in mental health care presents unique challenges, requiring a delicate balance between cost control, patient outcomes, and provider sustainability.
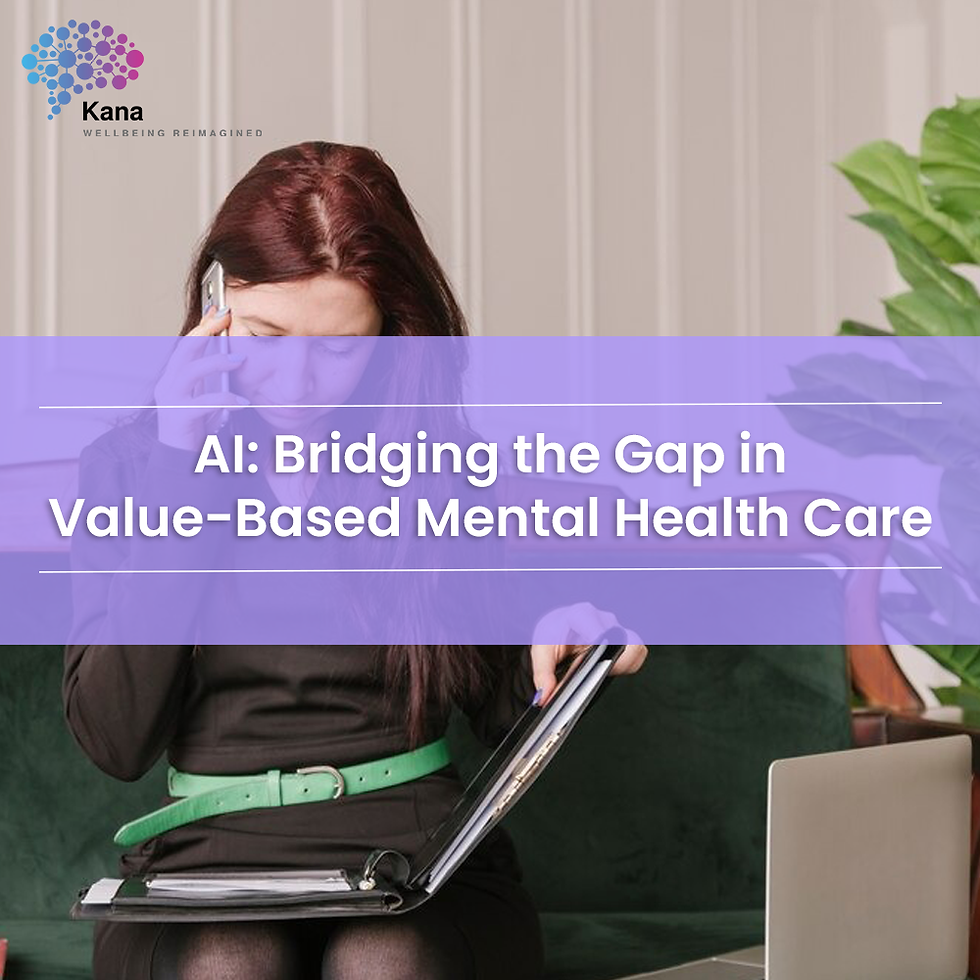
A powerful new tool—artificial intelligence (AI)—has emerged to help mental health providers navigate these challenges, transforming data into actionable insights and facilitating more personalized, proactive care. While integrating AI within VBC frameworks holds tremendous promise, certain hurdles must be addressed for widespread adoption.
The Rise of Value-Based Care in Mental Health
With the Centers for Medicare and Medicaid Services (CMS) aiming to transition all Medicare beneficiaries to VBC by 2030 (up from just 7% in 2021), VBC is reshaping healthcare. This shift demands robust data management to monitor patient outcomes, assess risks, and maintain care quality.But managing these vast data volumes is especially challenging in mental health care, where traditional outcome measures are not always clear-cut, and where patient progress may be slower or harder to quantify.
AI offers a promising solution by enabling mental health providers to measure, predict, and enhance patient outcomes while reducing the administrative burden of data management.
Risk Adjustment: Moving from Reactive to Proactive
Risk adjustment is essential in value-based care, ensuring that healthcare providers are adequately reimbursed based on the complexity of each patient’s health needs. Traditionally, risk adjustment has been a retrospective process, with data analyzed after the fact to secure accurate reimbursements. However, this approach can leave providers working with outdated information and hinder proactive patient care.
AI allows mental health providers to shift to a proactive risk adjustment model by integrating external data sources, such as pharmacy records, health exchanges, and out-of-network specialists. AI can create a comprehensive, real-time picture of each patient’s mental and physical health, allowing for timely intervention.
For example, if a patient with known diagnoses of depression and hypertension shows signs of additional mental health challenges, AI might flag these conditions based on new data, prompting providers to adjust treatment strategies before symptoms worsen.
Improving Patient Engagement and Communication
Effective communication is central to value-based care, as it promotes patient engagement and adherence to treatment plans. AI tools can personalize communication strategies based on individual preferences, making outreach efforts more effective. For example, AI might suggest that a patient prefers text-based reminders for appointments or follow-up care instructions.
By customizing communication, AI nurtures a stronger provider-patient relationship, increasing the likelihood that patients will stay engaged in their treatment plans. This is especially important in mental health care, where dropouts are common. Tailoring reminders and engagement strategies to patient preferences fosters a supportive environment that reduces dropout rates and supports continuous care.
Integrating AI for Preventive and Personalized Care in Mental Health
AI’s predictive capabilities are invaluable for preventive care, allowing providers to identify at-risk patients based on longitudinal data analysis. By tracking trends in patient data, AI can flag potential health risks, enabling providers to implement preventive measures.
For example, AI may identify a pattern of low mood during specific times of the year, prompting the provider to introduce coping mechanisms or preventive interventions, such as extra therapy sessions or lifestyle modifications, ahead of a potential depressive episode. This personalized approach ensures that care aligns with each patient’s unique needs, supporting better outcomes.
Realizing the Future of Value-Based Care with AI
AI’s integration into mental health care offers a powerful toolset that supports the goals of value-based care by improving diagnostic accuracy, facilitating early intervention, enhancing patient engagement, and reducing administrative burdens. As health systems and organizations adopt value-based models, AI can serve as a critical partner, enabling mental health providers to deliver cost-efficient, patient-centered care.
Kommentare